Leak Prevention in AI: Safeguarding Innovation and Trust
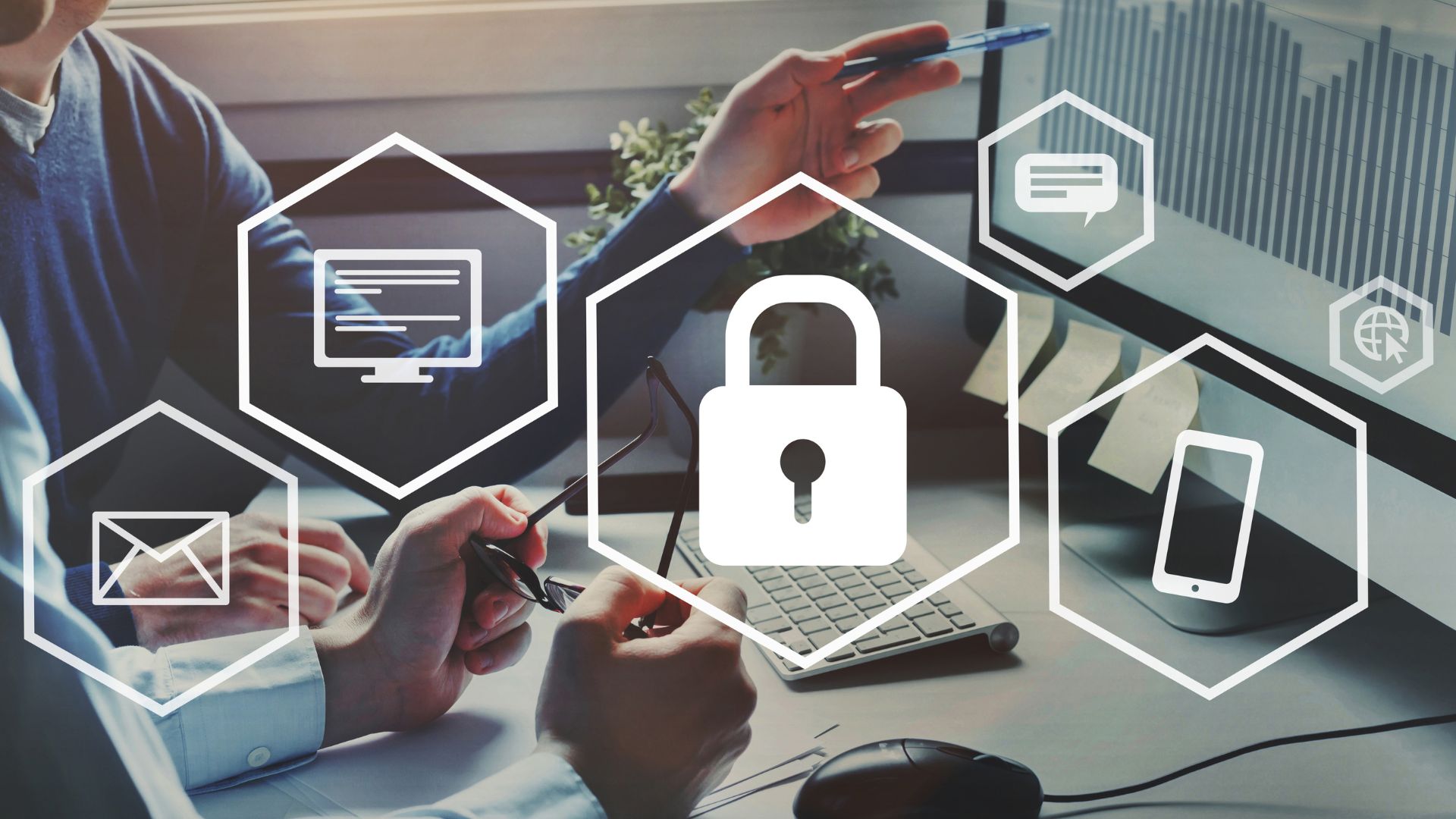
Introduction
In the age of artificial intelligence (AI), data is the lifeblood that fuels progress and innovation. However, with great power comes great responsibility. As the CEO, one of my top priorities is ensuring that the technologies we develop are not just powerful but also secure. Leak prevention in AI is not just a technical necessity—it is a cornerstone of trust, credibility, and sustainability in our industry. This blog delves into the importance of leak prevention in AI systems, the challenges we face, and the proactive measures we can adopt to safeguard our innovations and the data entrusted to us.
Why Leak Prevention Matters in AI
AI systems thrive on data. Whether it's sensitive customer information, proprietary algorithms, or intellectual property, any form of leakage can have severe consequences—financial, reputational, and ethical. A 2023 report by IBM highlights that the average cost of a data breach globally stands at $4.88 million, with AI and automation reducing breach lifecycle by nearly 108 days. However, the same AI-driven systems can also become vulnerable points if not designed with stringent safeguards.
Leak prevention isn't just about securing data; it's about maintaining the trust of your clients and users. A single breach can undo years of innovation and erode the confidence of stakeholders. For AI companies like ours, where intellectual property is our competitive edge, leak prevention is critical to sustaining long-term growth and success.
Challenges in Leak Prevention for AI Systems
The AI ecosystem faces unique challenges when it comes to preventing leaks:
1. Data Exposure Across Pipelines
AI projects often involve multiple stages—from data collection and preprocessing to model training and deployment. Each stage introduces potential vulnerabilities where data could be exposed.
2. Insider Threats
A 2022 study by Verizon Data Breach Investigations Report noted that insiders were responsible for 20% of breaches. Employees with access to sensitive data or code can unintentionally or maliciously cause leaks.
3. Third-Party Risks
Collaborations with vendors, cloud providers, or external teams expose AI firms to risks stemming from weak security practices outside the organization.
4. Model Inversion and Data Reconstruction
Attackers can exploit trained AI models to infer sensitive details about the training data—a process known as model inversion. For instance, in healthcare AI, such attacks could reveal private patient information.
5. Lack of Standardization
The rapid pace of AI innovation has outpaced the development of standardized frameworks for security, leaving us to navigate a fragmented and evolving landscape.
Strategies for Leak Prevention
At Bankai Labs, we believe in embedding security into the DNA of our AI systems. Here are the proactive measures we’ve implemented:
1. End-to-End Encryption
All data at rest and in transit is encrypted using state-of-the-art encryption protocols. This ensures that even if data is intercepted, it remains unreadable to unauthorized parties.
2. Secure Development Practices
Our developers adhere to secure coding practices, employing static and dynamic analysis tools to identify and mitigate vulnerabilities during development.
3. Access Control and Auditing
Role-based access controls (RBAC) limit data and system access to only those who absolutely need it. Comprehensive audit trails provide visibility into who accessed what, when, and why.
4. Continuous Monitoring
Real-time monitoring systems flag anomalies, unauthorized access attempts, or unusual activity in our pipelines, allowing us to respond swiftly to potential threats.
5. Vendor Risk Management
We evaluate the security practices of all third-party vendors and require adherence to strict data protection standards before collaboration.
The Future of AI Security
As AI systems become more integrated into critical industries, the stakes for leak prevention will only rise. Emerging technologies like blockchain could play a pivotal role in creating immutable records of data access and usage. Additionally, advancements in AI explainability and robustness testing will further enhance the ability to identify and mitigate risks proactively.
Many AI companies like ours must lead the charge, fostering a culture of security-first innovation. By collaborating with policymakers, industry leaders, and researchers, we can establish best practices and standards that protect the integrity of AI systems while promoting their widespread adoption.
Conclusion
Leak prevention in AI isn’t just about protecting data—it’s about protecting the trust and innovation that underpin the AI revolution. I’m committed to ensuring that our technologies are not just cutting-edge but also secure and reliable. By adopting a proactive and holistic approach to security, we can navigate the challenges of AI democratization while safeguarding the future of this transformative technology. Together, we can build a world where AI enhances lives without compromising safety or trust.
Get in touch
Reach out today for personalized assistance and tailored solutions. Contact us now for answers and a customized quote. Let's bring your vision to life efficiently and effectively!